Q&A: UCLA Health Chief Data Officer Albert Duntugan talks AI integration in health care
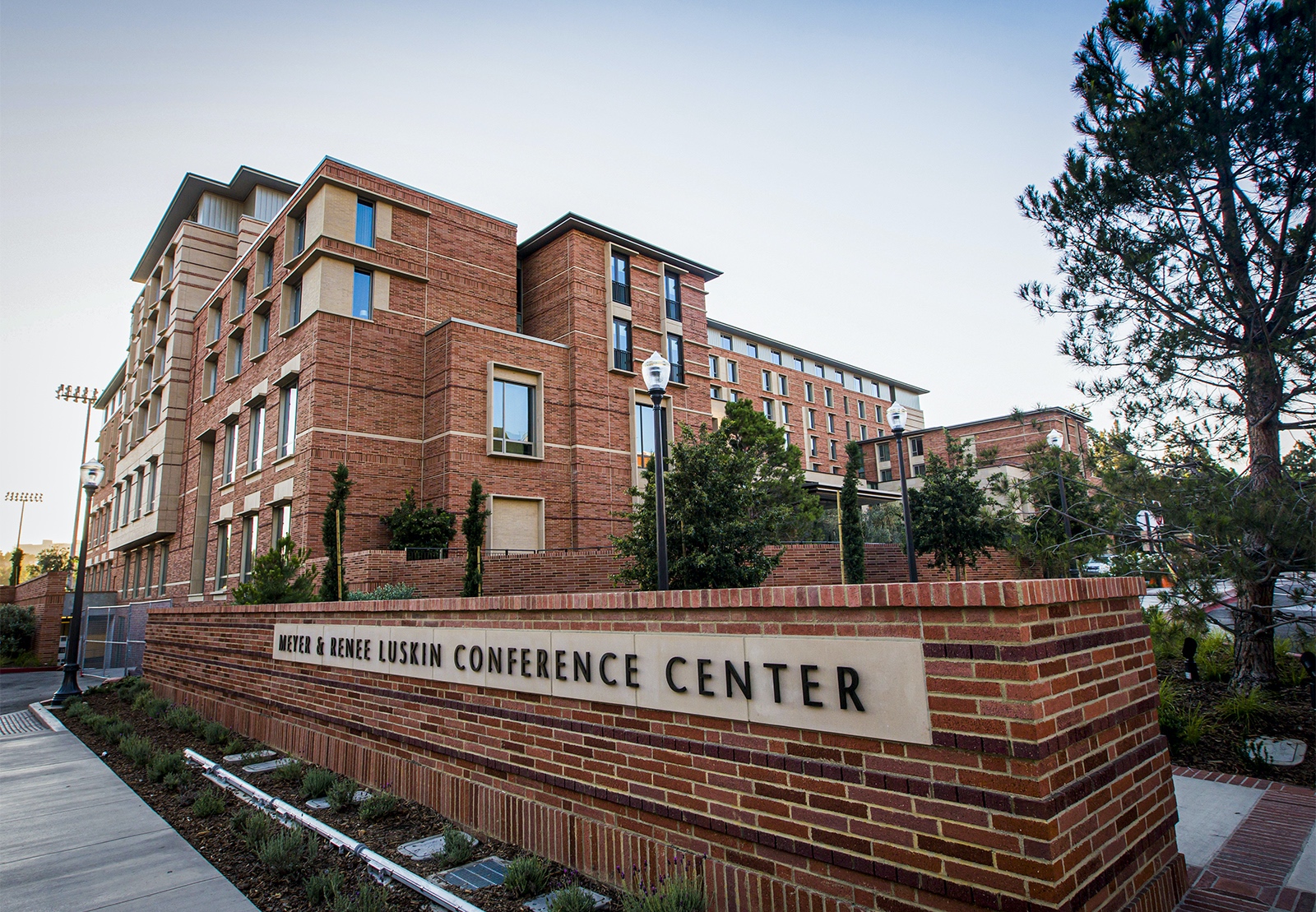
The annual Data Day event was held at the Meyer and Renee Luskin Conference Center. Many ideas that Albert Duntugan, one of the panelists, spoke about are working on being implemented. (Daily Bruin file photo)
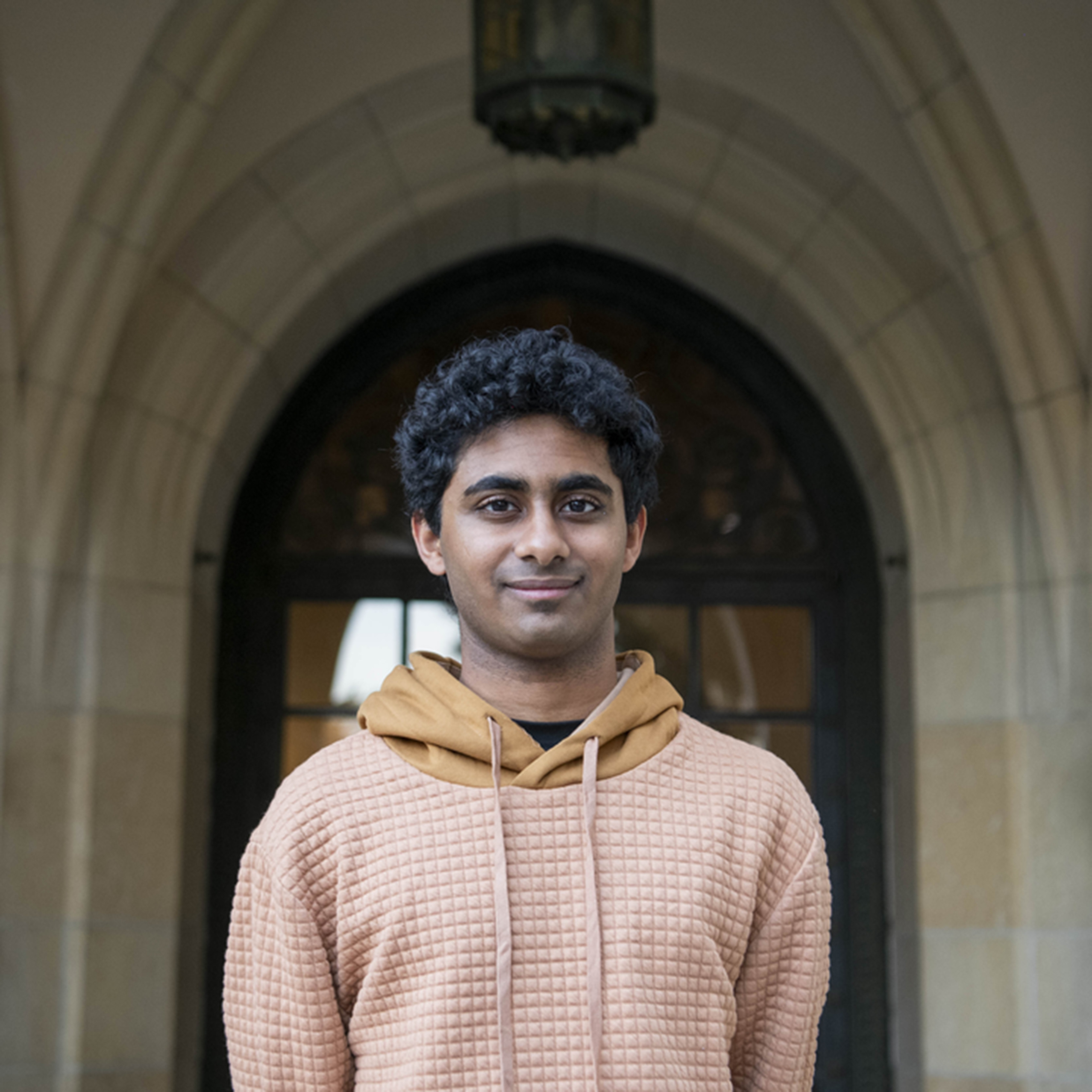
By Shaun Thomas
Dec. 5, 2024 8:44 p.m.
Albert Duntugan, the chief data officer of UCLA Health, sat down with Daily Bruin science and health editor Shaun Thomas to discuss UCLA’s Health’s plans to integrate artificial intelligence in its current health care network.
UCLA Health held its annual Data Day symposium June 4 at the Meyer and Renee Luskin Conference Center. Panelists from UCLA and other higher education institutions discussed topics ranging from addressing challenges related to voice AI to using special computing to tackle mental health challenges.
UCLA Health has been working on implementing many of these ideas relating to information technology and AI, Duntugan said.
This interview has been edited for length and clarity.
Daily Bruin: Given all the optimism surrounding AI and health care, what specific areas within UCLA Health are currently seeing the most impactful applications, and what are some areas you think AI just couldn’t replace yet?
Albert Duntugan: “Health AI” is a big term. If you look at the early use cases or the early problems we were trying to solve, we were proud of our ability to create our own custom way of doing natural language processing.
(An) example of using NLP technology is with patient messaging. So, when patients use our MyChart application on their smartphones, they can speak to their doctors. Sometimes their messages may have a high sense of urgency, so they may be saying, “I have this pain in my chest. It feels like an elephant is standing on me.” That’s a sign that they may be having a heart attack, but the patient may not know it.
Normally, we have about a dozen or so nurses in another unit – in what’s called an ambulatory care triage unit – that are looking through all of these messages. So by having our AI solution read these notes from the patients instead and making a prediction on whether it’s acute enough to have a nurse jump on that message – say, “Hey, you may really want to come into the emergency room right now,” – our AI solution is able to look through all the messages very quickly.
Can AI solve everything? Absolutely not. We can’t just lead with technology first. We have to know what is the problem in clinical workflow, in business workflow. It requires humans to know that. All technology is is a tool. That’s all AI is. It’s always humans leading the way.
DB: The panelists from Data Day also talked about concerns such as patient disclosure and patient consent. Could you elaborate more on how UCLA Health is going to handle disclosures about the use of AI in patient care?
AD: When working with our EHR (electronic health records) vendor, Epic, one of the things they recommend for the responsible use of AI is to have some kind of indicator within the user interface (that) lets a health care worker know whether AI was involved in the creation of a prediction or not.
The best practices that we’re seeing so far is to definitely have that indicator. AI is making a prediction and it says, “Hi, nurse or hi radiologist, I’m me, the AI. I made this prediction, and this is why I made it. These were the variables that led me to think that this is the real deal and that you should look at it, and it’s up to you, human, to decide whether you want to go about this or not.”
In terms of patient-facing AI, we don’t have many examples of that right now. We have one, but it’s in a proof-of-concept phase, where a physician’s messages to the patients are being auto-generated by ChatGPT. So, a patient will send a message to a doctor asking for something. The physician – instead of starting from scratch – will have a generated draft message response from Epic, from the machine, from AI saying, “This is what I would say.” Then, a physician needs to now modify the note, or they send it out as is.
DB: How is UCLA Health also planning to leverage these language models beyond things such as scribing or patient follow-ups or even clinical workflow?
AD: The fun thing about working at UCLA Health is we’re a major academic health system, and part of our job is to push the boundaries of clinical research. We see large language models being used in research where an investigator would be able to take notes from (an) electronic health care record or any kind of unstructured text and run it through an LLM to … extract information that’s inherent in the text.
An effort you’ll see in the medical informatics space is to have terminology in order to explain clinical concepts like diagnoses or procedures. So there are many terminologies where we have some kind of alphanumeric code to describe an event, like seeing a brand new patient in your physician office or giving a patient an x-ray.
There’s a code to explain that procedure. So these are codes, but humans don’t talk in codes, we talk in regular language. But in order to process data, it’s very useful for us to have codes. So we can use the LLMs (Language Learning Models) to read through all of this text and translate concepts; it’s called information extraction or IE.
In this case, we wouldn’t just be using ChatGPT from OpenAI and the Microsoft folks. We could be using LLMs from Meta like Llama. We would pull it from Hugging Face and other communities where this is available, and we would go ahead and turn them on here locally at UCLA in a very safe and secure environment where patient privacy is of the highest concern.
DB: Overall, what role do you see UCLA Health playing in the broader health care AI community?
AD: UCLA Health and the other UCHealth sites collectively: We are the University of California Health. I see us playing a very big role. We already have a seat at the table for what’s called the Coalition for Health AI or CHAI. It’s an organization made up of a number of academic health systems and other important organizations in health care. It has connections to the government and public sector space as well.
It’s nice to have that seat at the table, but within this forum and others like it, we’re addressing a lot of these issues for handling AI responsibly.
Another is within our own wonderful University of California. We have an AI Council and I’m a member of that. We provide input into how AI is used not just in health care but also in our teaching mission as well, so education and the use of AI at all of our campuses.
We’re looking at general concerns like when it’s time to procure AI, an AI solution or buy an AI solution from a vendor, what are the steps that we should take to evaluate the risk and security? How do we balance issues of cost, too, if all this stuff is going to (be) expensive?
There’s also a UC Health AI Governance Forum, where we just focus only on the UC Health, all six of the campuses that have a health care clinical practice going.
DB: Do you have any advice for UCLA students who want to follow your path or even incorporate big data into their work, especially those interested in health care as well?
AD: I’ll say three things. One is “Be curious.” You may not be in health care now. You’re in school, and you’re studying whatever you need to study. If you’re curious about AI, use it. Go to the UCLA GenAI website, go ahead and use the Bing interface or the ChatGPT interface and see how it can affect your studies.
Is ChatGPT really helping you? Are there aspects of prompt engineering that you need to learn to inject it into your educational workflow, into to your studies? A lot of this technology is sitting out there raw, and that means rolling up our sleeves and getting our hands dirty.
Second thing is if you’re interested in health care, there are products from OpenAI and other vendors where they’re facing health care directly. There are studies where they’re using de-identified data, where you can pull de-identified datasets from other organizations and you can play with it. If you’re into programming, definitely keep pursuing that. You may have a major where you have to do that already.
Finally, as great as those basics are, the real world is so different. When you work at UCLA Health, someone doesn’t give you a clean set of data. It’s very messy – there’s a lot of interactions with other stakeholders, so you’re practicing skills beyond just being a brainy scientist.
You’re working on your communication skills, your appreciation for business and for how a health system works. That’s where these internship opportunities are very important. I would argue that when you’re in school, you need to do as many internships as possible before you graduate and enter the workforce. This can only help you, and I know it’s hard to get those internships, but I like to say I’m doing my part by running one program of many in the country to make those opportunities possible.